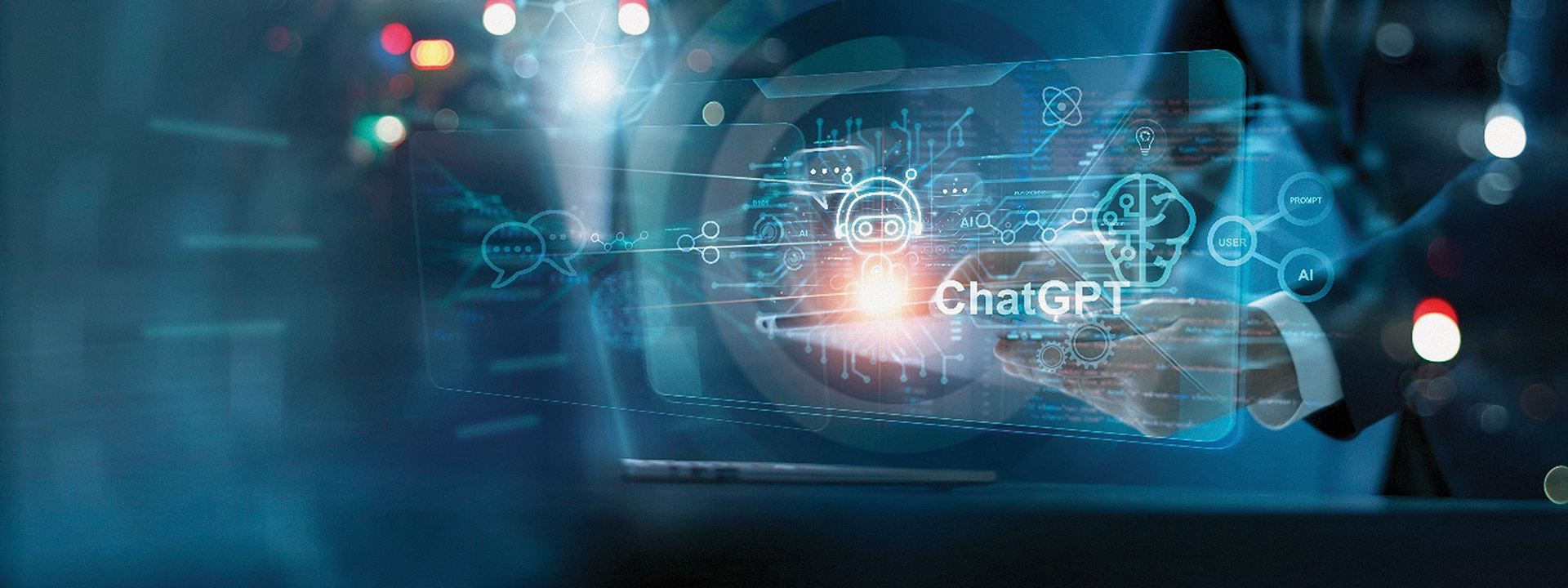
Artificial Intelligence (AI) has become a ubiquitous buzzword in today’s technological landscape, with its applications ranging from voice assistants to self-driving cars. The concept of AI has now gone through several stages of evolution to become what it is today. The origins of AI date back to the mid-20th century when computer scientists began exploring the possibility of machines that could perform tasks that required human intelligence. One of the first examples of AI was the ‘Logic Theorist’, developed in 1955 by Allen Newell and Herbert A Simon, which was a programme that could prove mathematical theorems.
The 1960s saw the emergence of symbolic AI, which relied on the manipulation of symbols and rules to perform tasks. One of the notable examples of symbolic AI was the General Problem Solver (GPS), developed by John McCarthy and Marvin Minsky in 1961, which could solve a wide range of problems by representing them as symbolic structures. In the 1980s, AI experienced a paradigm shift with the introduction of connectionist AI, also known as neural networks. Neural networks mimic the structure and function of the human brain and are capable of learning from data. The development of backpropagation, a technique for training neural networks, led to breakthroughs in fields such as image and speech recognition.
The 1990s saw the emergence of statistical AI, which combined elements of symbolic AI and connectionist AI. Statistical AI relies on probabilistic models to make predictions and decisions based on data. This approach has been successful in areas such as natural language processing and recommendation systems. In recent years, AI has experienced another significant shift with the rise of deep learning, which allows AI systems to learn and improve from vast amounts of data. Deep learning has led to breakthroughs in areas such as image and speech recognition, natural language processing, and predictive analytics. Today, deep learning algorithms power many AI applications, including self-driving cars and medical diagnosis.
The evolution of AI has been driven by advancements in computing power, algorithms, and data availability. The emergence of cloud computing has made it easier and more cost-effective for businesses and organisations to implement AI solutions. The availability of big data has also been a game-changer, allowing AI systems to learn and improve from vast amounts of data. The evolution of AI has been marked by several significant shifts, from symbolic AI to neural networks and deep learning. The future of AI is likely to be driven by advancements in areas such as explainable AI, which aims to make AI systems more transparent and understandable, and reinforcement learning, which allows AI systems to learn from feedback and experience.
As AI continues to evolve, its potential to transform industries and disrupt traditional business models will only grow. It has become a critical component for organisations and businesses to stay competitive and relevant in today’s fast-paced, data-driven world. However, implementing AI in an organisation can be a daunting task, and many businesses struggle to make it work effectively. Here, we will discuss some key steps that businesses can take to successfully implement AI in their operations.
Before implementing AI in your organisation, you need to identify the problem that you want to solve. This can range from automating routine tasks to improving customer experience. Once you have identified the problem, you can start looking for AI solutions that can help you achieve your goals.
There are several AI technologies available in the market such as machine learning, natural language processing, and computer vision. It is essential to choose the right technology that aligns with your business objectives and solves the problem you have identified. AI algorithms require vast amounts of data to learn and make predictions. Therefore, it is critical to gather and prepare data that is relevant and accurate. Data preparation involves cleaning, organising, and labelling data to make it suitable for AI analysis.
Once you have gathered and prepared data, you need to develop and train AI models. This involves selecting the appropriate algorithm, configuring the parameters, and training the model on the data set. It is essential to evaluate the model’s performance regularly and refine it based on feedback. After developing and training the AI model, it is time to integrate it into your operations. This involves deploying the model in a production environment and integrating it with your existing systems and processes. You may also need to train your employees to use AI tools and systems effectively.
It is essential to monitor and evaluate AI performance regularly. This involves measuring the accuracy of the model’s predictions, identifying areas for improvement, and refining the model accordingly. You may also need to retrain the model periodically to keep up with changing business needs. As with any technology, AI raises ethical and legal concerns, such as data privacy, bias, and accountability. These issues must be addressed upfront and AI design systems must be transparent, fair and accountable.
With significant AI progress in recent years, it has had many subsequent positive advancements and benefits across industries. However, like any technology, AI also poses certain risks that must be addressed to ensure its safe and responsible development and use. Here are some of the possible risks of AI and how they can be mitigated. AI algorithms learn from data, and if the data contains biases, it can lead to biased decisions and discrimination. For example, if an AI algorithm is trained on historical hiring data that contains biases against certain demographics, it may perpetuate those biases in the future. To mitigate this risk, it is essential to ensure that the data used to train AI models is diverse and representative of the population.
As AI systems automate tasks previously performed by humans, there is a risk of job loss and unemployment. This is particularly concerning for low-skilled jobs that are vulnerable to automation. To mitigate this risk, it is essential to invest in reskilling and upskilling programmes to help workers transition to new roles that require human skills and expertise. AI systems can be vulnerable to cyberattacks and data breaches, which can compromise sensitive information and cause significant harm. To mitigate this risk, it is essential to implement robust security protocols and conduct regular security audits to identify and address vulnerabilities.
Autonomous weapons systems that can make decisions without human intervention pose a significant risk of accidental or intentional harm. To mitigate this risk, it is essential to regulate the development and use of autonomous weapons and ensure that they are subject to human oversight and control. As AI systems become more integrated into our daily lives, there is a risk of over-reliance and dependence on them. This can lead to reduced critical thinking and decision-making skills, which can be harmful in situations where AI systems fail or make errors. To mitigate this risk, it is essential to maintain human decision-making and oversight over AI systems.
AI systems can process vast amounts of personal data, raising concerns about privacy and data protection. To mitigate this risk, it is essential to implement strong data protection regulations and ensure that AI systems comply with them. Additionally, organisations should be transparent about the data they collect and how it is used. AI offers many benefits, but it also poses certain risks that must be addressed to ensure its safe and responsible development and use. To mitigate these risks, it is essential to implement robust regulations, invest in ethical and responsible AI development, and ensure that human decision-making and oversight are maintained over AI systems. By doing so, we can maximise the benefits of AI while minimising its potential harms.
READ ALSO:
Published Date: June 1, 2023, 12:00 am
Post Comment
E-Magazine
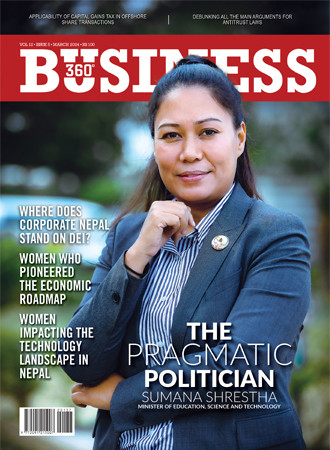
Click Here To Read Full Issue
RELATED BitsNBytes